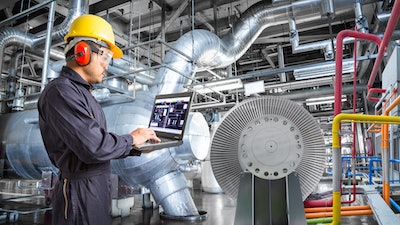
Across the process industries, advanced analytics is a problem-solving journey through historical, current and predicted time series data, with insights driving improved decision-making.
Over the past few decades, process engineering teams began leveraging stored data to inform process changes focused on preventive maintenance, mitigation strategies and optimization initiatives for improving operational efficiency. These efforts began with a focus on monitoring, concentrating on the most recent operations data available to identify issues. As these explorations continued, organizations dove into the data stored in historians or other databases to fine-tune insights, using diagnostic analytics to investigate recent issues, such as a deviation during the previous batch.
To achieve the highest efficiency possible, today’s process manufacturers must shift from reactive approaches that address issues as they arise to a proactive approach, leveraging historical data and context to better inform process improvement decisions. Fortunately, modern advanced analytics applications enable these manufacturers to implement proactive solutions that anticipate problems before they occur, leading to greater efficiency and performance.
The Basics of Analytics
“Analytics” is a broad-brush term, increasingly associated with software products, platforms and the cloud. Therefore, it must be qualified with modifiers. For example, advanced analytics describes the use of statistics and machine learning in analytics to assess and improve insights. Other modifiers can be used to differentiate the analytics type based on utility and complexity.
Diagnostic analytics uses information from past events to respond to a given situation, examining a raw set of historical data and applying statistical analysis to identify patterns and gain insights. Using this approach, teams are faced with an inescapable lag between the event or issue under analysis and the action taken to improve future performance, eliminating the ability to prevent damage outright.
Similarly, descriptive analytics also looks at the past, with the goal of summarizing events via reports that personnel can easily interpret and learn from. In descriptive analytics, the same lag occurs and it can be difficult to develop clear-cut answers on how and when to make decisions that will prevent future performance issues.
While both of these retrospective methods provide value, they fail to solve the whole puzzle. Predictive analytics provides the missing piece by leveraging historical data to build and train models that create a projection of future data, enabling teams to anticipate what will happen, when it will happen and the actions operators should take to impact desired outcomes.
Advanced Analytics in Maintenance
Before predicting future system behavior, an engineer must understand past and present operating conditions. This is best achieved through live connections with all relevant process and contextual databases.
Manufacturing organizations can leverage advanced analytics applications to connect disparate data sources to a central cloud-based source, alleviating the challenges of live data connectivity, such as retaining multiple logins and constantly bouncing from one interface to another. These applications provide process experts and engineers with simplified data-cleansing tools and contextualization, empowering them to quickly derive meaningful and reliable insights from all available data.
Once live connections are made, teams can embark on the journey to valuable predictions, including equipment-based predictions—or predictive maintenance—where the goal is to anticipate when equipment will require maintenance or fail. Predictive maintenance techniques vary, but the objectives of increasing quality, reliability and uptime are fixed.
An advanced analytics application’s predictive capabilities are driven by advanced algorithm functionality, in which the application matches an organization’s specific workflows and procedures to a respective law, theorem, or process design principle to generate key performance indicators. This enables accurate data extrapolation when expanding future time frames for insight and analysis.
Results
Developing a maintenance strategy built on predictive analytics has transformed operations across the process industries, as demonstrated in the use cases that follow.
Filter membrane predictive maintenance
Filter membranes—which are found throughout the process and utility sides of pharmaceutical, chemical and food and beverage plants—foul and degrade over time, requiring maintenance to clean.
A major pharmaceutical manufacturer plagued by unexpected fouling rates went looking for a method to predict when membrane replacement would be required. Using Seeq, an advanced analytics application, the manufacturer applied Darcy’s Law to calculate the filter membrane resistance based on pressure and flow sensor data, as well as known values of surface area and fluid viscosity. By reducing the variables of interest to just these few, engineers identified patterns in the data of decaying membrane performance (Figure 1).
Figure 1: By using Seeq to reduce the variables of interest for multiple filtration membrane sensors, a manufacturer identified patterns of fouling and used these to project appropriate maintenance schedules.Seeq
By extrapolating the model into the future, the manufacturer could predict fouling rates and schedule maintenance activities accordingly, maximizing the lifespan of the filters while providing minimal impact on operations.
Compressor health monitoring and maintenance
Detecting and predicting compressor performance issues, which can lead to catastrophic shutdown of operations or issues with environmental safety, is another common use case for predictive maintenance. Advanced analytics can be used to monitor compressor health variables to detect poor performance or mechanical degradation without tedious time spent examining data in spreadsheets.
In one large manufacturing facility, data scientists used an advanced analytics application with machine learning algorithms to determine the root cause of compressor failure. Next, they superimposed the algorithm on live data to point out degrading performance and provide maintenance notifications to mitigate failure, with insights provided in a visual interface to operators and process engineers.
These types of tools empower engineers to identify leading and lagging indicators of compressor health and continuously monitor variables, helping teams proactively identify risks and prioritize maintenance activities.
Look Back to Move Forward
Making accurate process manufacturing predictions relies on in-depth knowledge of past equipment behavior and outcomes. By using advanced analytics applications to combine retrospective with predictive analytics, process experts and data analysts can easily build robust models, capable of predicting plant maintenance needs and risk-mitigating procedures.
With the right tools in their digitalization toolbox, process manufacturers can build better models to provide vast plant insights. This helps project equipment issues prior to failure so personnel can optimize maintenance schedules and prevent costly downtime.
---
Joe Reckamp is an Analytics Engineering Group Manager at Seeq Corporation. He enjoys working with engineers across manufacturing industries to improve processes and realize value using process data analytics. He received his B.S. and M.S. in Chemical Engineering from Villanova University, working in the pharmaceutical industry throughout most of his career, including stints in research and development with GlaxoSmithKline and production with Evonik.